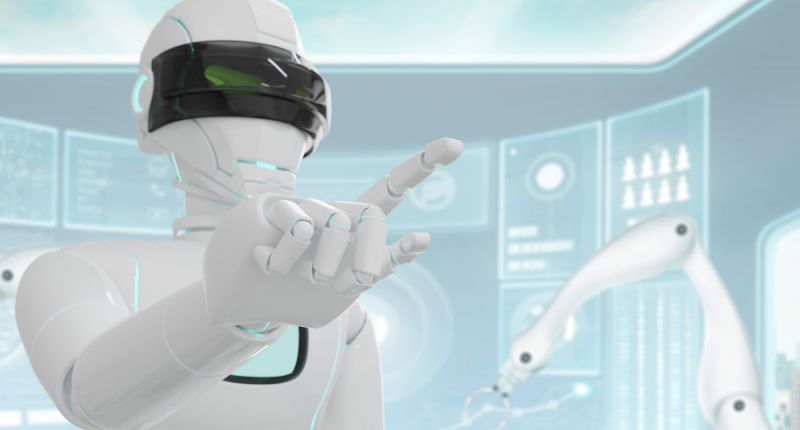
How do ai detectors work? Artificial Intelligence (AI) detectors have become integral in our modern technological landscape, shaping industries and influencing our daily lives. But how do AI detectors work, and what makes them such powerful tools? In this in-depth exploration, we will demystify the mechanics behind AI detectors, unraveling the complexities to provide a comprehensive understanding.
Table of Contents
Understanding the Basics of AI Detectors
Definition and Purpose
AI detectors, at their core, are systems designed to recognize and interpret patterns or anomalies within data. They play a pivotal role in various industries, from enhancing security measures to revolutionizing healthcare diagnostics.
Historical Evolution of AI Detectors
The evolution of AI detectors traces back to the mid-20th century, with significant advancements in sensor technologies and machine learning algorithms driving their development.
Significance in Various Industries
Industries such as security, healthcare, and automotive have embraced AI detectors for their ability to process vast amounts of data swiftly and accurately, leading to improved decision-making processes.
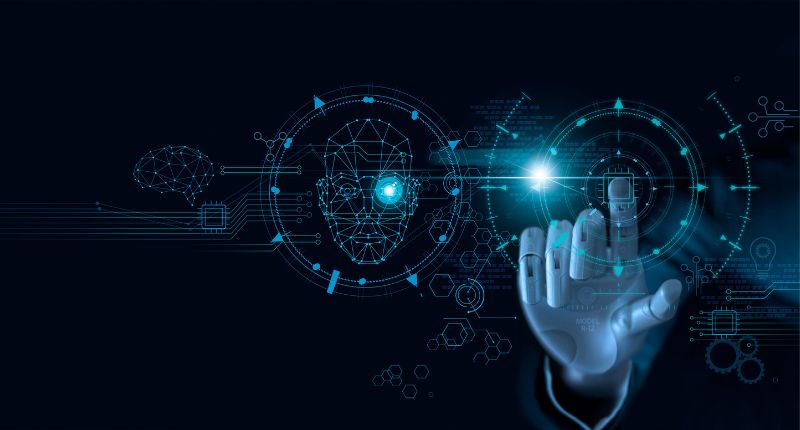
The Core Components of AI Detectors: How Do AI Detectors Work
Sensor Technologies
- Types of Sensors Used
- Infrared sensors for thermal imaging.
- Lidar sensors for precise distance measurements.
- Audio sensors for detecting sound patterns.
- Sensing Modalities
- Visual data for image recognition.
- Auditory data for sound classification.
Data Collection and Processing
- How Data is Gathered
- Through sensors, capturing information from the environment.
- Integration with IoT devices for comprehensive data sets.
- Pre-processing Steps
- Cleaning and filtering data for accuracy.
- Normalizing data to ensure consistency.
Feature Extraction
- Identifying Key Data Points
- Algorithms focus on extracting relevant features.
- Reducing data dimensionality for efficient processing.
- Transforming Raw Data into Usable Information
- Converting raw sensor data into actionable insights.
- Creating a foundation for machine learning algorithms.
The Role of Machine Learning in AI Detectors
Overview of Machine Learning Algorithms
Machine learning empowers AI detectors through algorithms capable of learning patterns from data and making predictions or decisions based on that learning.
Training AI Detectors: How Do AI Detectors Work
- Supervised Learning
- Training with labeled data for accurate predictions.
- Common in image and speech recognition.
- Unsupervised Learning
- Clustering and association for pattern discovery.
- Useful in anomaly detection.
Fine-Tuning for Improved Accuracy
- Continuous Learning
- Adapting to new patterns in real-time.
- Ensuring detectors stay relevant and accurate.
- Adaptability to New Patterns
- Incorporating feedback loops for ongoing improvement.
- Enhancing accuracy through continuous refinement.
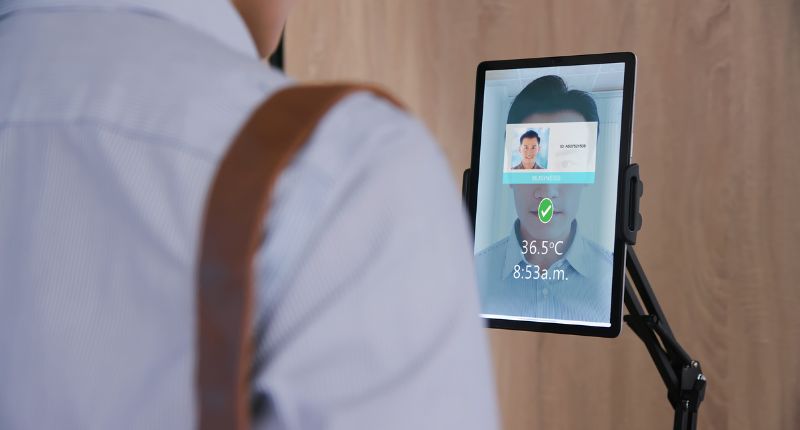
Common Applications of AI Detectors
Security and Surveillance
- Facial Recognition
- Enhancing security through biometric identification.
- Widely used in airports, border control, and law enforcement.
- Intrusion Detection
- Protecting networks by identifying unusual activities.
- Crucial for safeguarding sensitive data.
Healthcare
- Disease Diagnosis
- Analyzing medical images for early disease detection.
- Assisting healthcare professionals in accurate diagnoses.
- Patient Monitoring
- Continuous monitoring of patient vitals for timely interventions.
- Improving patient care and outcomes.
Automotive Industry
- Collision Avoidance Systems
- Utilizing sensors for real-time detection of obstacles.
- Enhancing driver safety.
- Driver Assistance Technologies
- Adaptive cruise control and lane-keeping systems.
- Transforming the driving experience.
Challenges and Ethical Considerations
Bias in AI Detectors
AI detectors may inherit biases present in training data, leading to discriminatory outcomes. Addressing bias is crucial for equitable and fair applications.
Privacy Concerns
As AI detectors often involve the analysis of personal data, ensuring robust privacy measures is essential to maintain public trust.
Transparency and Accountability
Establishing clear guidelines for the functioning of AI detectors ensures accountability and transparency in their decision-making processes.
Real-world Examples and Success Stories
In the ever-evolving realm of AI detectors, real-world applications showcase the transformative impact these systems have on various industries. Let’s delve into some compelling examples and success stories that illustrate the power and versatility of AI detectors.
1. Predictive Policing: Reducing Crime Rates
Case Study: Los Angeles Police Department
The Los Angeles Police Department (LAPD) implemented AI detectors to enhance their crime-fighting strategies. By analyzing historical crime data, the system identifies patterns and predicts potential crime hotspots. This proactive approach has resulted in a significant reduction in crime rates, demonstrating the potential of AI detectors in augmenting traditional policing methods.
2. Medical Imaging: Precision in Disease Diagnosis
Case Study: IBM Watson Health
IBM Watson Health has been at the forefront of leveraging AI detectors in medical imaging. Through machine learning algorithms, these detectors analyze medical images to aid clinicians in disease diagnosis. This technology has substantially improved the accuracy of identifying conditions such as cancer, enabling early interventions and ultimately saving lives.
3. Autonomous Vehicles: Collision Avoidance Systems
Case Study: Tesla Autopilot
Tesla’s Autopilot system integrates AI detectors for real-time analysis of the vehicle’s surroundings. Using a combination of cameras, radar, and ultrasonic sensors, the system can detect obstacles, pedestrians, and other vehicles. This technology forms the foundation for Tesla’s advanced driver-assistance features, contributing to safer driving experiences and a step closer to fully autonomous vehicles.
4. Facial Recognition: Enhancing Security Measures
Case Study: Airports and Border Control Systems
Airports and border control agencies worldwide have embraced facial recognition technology powered by AI detectors. These systems match facial features against databases of known individuals, enhancing security measures. The speed and accuracy of these detectors have revolutionized the process of identifying and apprehending individuals with security concerns.
5. E-commerce: Fraud Detection and Prevention
Case Study: Amazon Fraud Detector
In the dynamic world of e-commerce, AI detectors play a crucial role in fraud detection and prevention. Amazon, for example, employs AI detectors that analyze user behavior, transaction patterns, and other variables to identify potentially fraudulent activities. This not only safeguards customers but also ensures the integrity of the online marketplace.
6. Smart Cities: Traffic Management and Optimization
Case Study: Singapore’s Smart Traffic Management
Singapore’s Smart Nation initiative incorporates AI detectors in its traffic management systems. By analyzing real-time data from sensors and cameras, the system optimizes traffic flow, reduces congestion, and enhances overall urban mobility. This application showcases the scalability of AI detectors in creating smarter and more efficient urban environments.
7. Retail: Personalized Shopping Experiences
Case Study: Alibaba’s AI Shopping Assistant
Alibaba utilizes AI detectors to personalize the shopping experience for users. By analyzing past purchasing behavior and preferences, the system recommends products tailored to individual users, creating a more engaging and customer-centric e-commerce platform. This not only improves user satisfaction but also contributes to increased sales.
8. Environmental Monitoring: Precision Agriculture
Case Study: John Deere’s AI-powered Tractors
John Deere integrates AI detectors into its tractors for precision agriculture. These detectors analyze data from various sensors, such as soil moisture and crop health monitors, to optimize planting, irrigation, and harvesting processes. This sustainable approach not only maximizes crop yield but also minimizes environmental impact.
In these real-world examples, AI detectors have proven to be versatile tools, offering solutions to complex challenges across diverse industries. As technology continues to advance, these success stories underscore the limitless potential of AI detectors in reshaping our world and driving innovation to new heights.
Future Trends and Innovations in AI Detectors
The field of AI detectors is dynamic, continuously evolving with technological advancements. As we peer into the future, several trends and innovations are poised to shape the landscape of AI detectors, propelling them into new realms of sophistication and utility.
1. Advancements in Sensor Technologies
Future AI detectors will likely benefit from strides in sensor technologies, marking a departure from traditional methods. Here’s what to anticipate:
- Enhanced Resolution: Sensors with higher resolution will provide more detailed and accurate data, improving the precision of AI detectors.
- Multi-Modal Sensors: Integration of multiple sensor types, such as combining visual and auditory sensors, will enable detectors to gather diverse and complementary information.
2. Integration with IoT and Big Data
The fusion of AI detectors with the Internet of Things (IoT) and Big Data analytics is a paradigm shift that promises transformative outcomes:
- Comprehensive Data Insights: AI detectors, when integrated with IoT devices, will have access to a wealth of real-time data, enabling more accurate and timely decision-making.
- Predictive Analytics: By leveraging Big Data, AI detectors can move beyond reactive responses, delving into predictive analytics for anticipating and preventing issues.
3. Quantum Computing and Neuromorphic Computing
The exploration of quantum computing and neuromorphic computing holds immense potential for revolutionizing the capabilities of AI detectors:
- Quantum Computing: The quantum realm offers unprecedented computational power, allowing AI detectors to process vast datasets at speeds unimaginable with classical computers.
- Neuromorphic Computing: Drawing inspiration from the human brain, neuromorphic computing aims to develop AI detectors with cognitive capabilities, enabling more nuanced and context-aware decision-making.
4. Explainable AI (XAI)
As AI detectors become increasingly complex, the need for transparency in their decision-making processes grows. Explainable AI is a trend focused on making AI systems more understandable:
- Interpretable Models: Future AI detectors will prioritize models that humans can interpret, fostering trust and facilitating collaboration between AI and human operators.
- User-Friendly Interfaces: Efforts will be directed towards creating interfaces that convey the reasoning behind AI decisions in a clear and accessible manner.
5. Edge Computing for Real-time Processing
With the rise of edge computing, the processing power of AI detectors is expected to move closer to the data source:
- Reduced Latency: Real-time processing at the edge will minimize latency, crucial for applications like autonomous vehicles and critical infrastructure security.
- Enhanced Privacy: Edge computing allows data to be processed locally, addressing privacy concerns associated with transmitting sensitive information to centralized servers.
6. Ethical AI and Bias Mitigation
As the deployment of AI detectors expands, addressing ethical concerns and mitigating bias in their decision-making processes becomes paramount:
- Ethical Frameworks: Future AI detectors will be developed with clear ethical guidelines, ensuring responsible and fair use.
- Bias Detection and Correction: Ongoing efforts will focus on creating algorithms that can detect and correct biases, promoting inclusivity and equity.
7. Robust Cybersecurity Measures
With the increasing integration of AI detectors in critical systems, ensuring their cybersecurity becomes a crucial consideration:
- Adversarial Defense Mechanisms: AI detectors will be equipped with advanced defenses against adversarial attacks, securing their integrity and functionality.
- Continuous Monitoring: Systems will implement continuous monitoring for potential security threats, safeguarding against malicious manipulation.
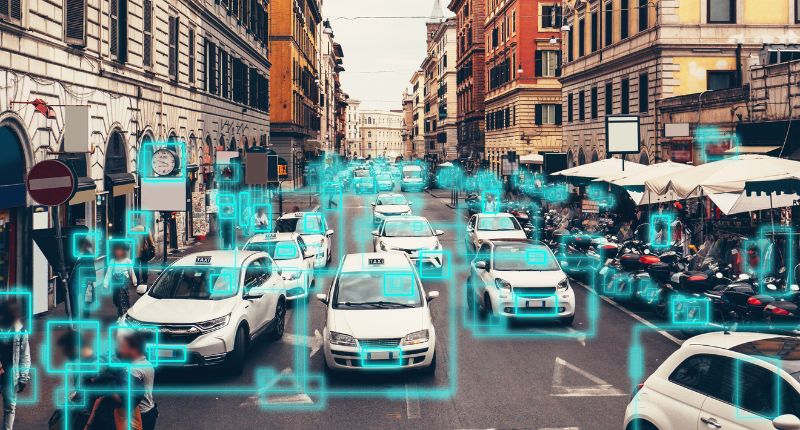
How Readers Can Leverage AI Detectors
Choosing the Right AI Detector for Specific Needs
- Identifying Use Case Requirements
- Understanding the specific application of AI detectors.
- Considering Sensor Modalities
- Matching sensor capabilities with detection needs.
Best Practices for Implementation
- Ensuring Data Privacy
- Implementing encryption and secure data storage practices.
- Complying with relevant data protection regulations.
- Regular System Updates
- Keeping AI detectors up-to-date with the latest algorithms.
- Addressing security vulnerabilities promptly.
Potential Benefits and Pitfalls
- Benefits
- Increased efficiency and accuracy in various processes.
- Enhanced decision-making capabilities.
- Pitfalls
- Potential for biases and ethical concerns.
- Overreliance without human oversight.
Conclusion
In conclusion, the intricate workings of AI detectors involve a combination of advanced sensor technologies and sophisticated machine learning algorithms. As we navigate the evolving landscape of AI, understanding these mechanisms becomes pivotal for informed decision-making and responsible use.
Additional Resources and References
For those eager to delve deeper into the world of AI detectors, the following resources provide valuable insights:
FAQs Section
How does AI content detector work?
AI content detectors utilize machine learning algorithms to analyze patterns and features within data. They are trained on labeled datasets, allowing them to make predictions or decisions based on new, unseen data.
Are AI detectors actually accurate?
The accuracy of AI detectors depends on various factors, including the quality of training data, the sophistication of algorithms, and continuous fine-tuning. In many applications, AI detectors can achieve high levels of accuracy.
How can AI use be detected?
Detecting AI use involves examining patterns of behavior, such as rapid and precise data analysis, autonomous decision-making, and the application of machine learning algorithms. Unusual or highly efficient processes may indicate AI involvement.
How to trick AI content detectors?
Attempting to trick AI content detectors is not encouraged, as it may lead to unintended consequences and undermine the purpose of these systems. AI detectors are continually evolving to identify and adapt to new patterns, making it challenging to deceive them consistently.